Disease control
Plant disease, regardless of its abiotic or biotic nature, can be defined as “anything that prevents the plant from performing to its maximum potential”, reducing its economic or aesthetic value [1]. Biotic agents refer to microorganisms and parasitic plants that usually are called pathogens. The nonliving environmental and nutritional factors and chemical substances, on the other hand, are called abiotic agents[2]. Plant disease will occur if all the following unfavorable conditions are satisfied simultaneously. I) the plant becomes susceptible to disease for any reason, such as nutrition deficiency and toxicity. II) the plant pathogens, including dormant or newly transferred ones, are present in the vicinity. III) the environmental condition is favorable for the pathogens’ activity[1].
Lucas and Campbell classified disease management methods into three main categories: prevention, genetic resistance, and chemotherapy[2]. Prevention can be achieved by methods such as proper site selection, planting date, or even proactive chemical application, to prevent the satisfaction of at least one of the three conditions mentioned. Genetic resistance management, which attracts increasing attention, is to identify the resistance sources genes in the plants and breed them to be naturally immune to a specific disease (will be discussed more in phenotyping section)[3]. Chemotherapy or using synthetic chemicals is one of the oldest and, nowadays, the most widely used methods for disease control in plants that has been practiced for over a century[4]. Although synthetic chemicals have been promising for food security and economic benefits, studies show that farmers tend to use more pesticides than needed [5]. Excessive use of pesticides caused various emerging issues and severe side effects[6], [7]. Hazardous effects of excessive pesticide usage can be classified as 1) the direct impact on humans, 2) impact through food commodities, 3) impact on the environment, 3) surface and groundwater contamination, 5) soil contamination and its effects on fertility, and 6) air contamination[8]. Usually, when a new pesticide is introduced, it takes several years for repercussions to appear in the environment, which explains why most of the lavishly practiced pesticides become prohibited later and goes to the long list of prohibited pesticides that are enforced by law[9]. Moreover, excessive use of some pesticides makes pathogens become completely resistant to that agent or decrease sensitivity[10].
Precision Pest Management (PPM) can help to ameliorate the hazards of excessive pesticide applications. PPM that is considered a subset of PA can be defined as the application of the right amount of pesticide, at the right place in the field, and at the right time[11]. Two main steps of PPM are I) using remote sensing techniques (mainly aerial imagery) to obtain site-specific information, and II) providing localized solutions using variable rate applications[12]. The first step, which is similar to other PA practices, uses the sensing methods discussed in the sensors section. RGB cameras are widely used for visually detectable disease and pests where leaf color changes due to a reduction of pigments that absorb light, thus increasing reflectance in specific bands of the visible region [13]. Multispectral sensors are suitable when the disease affects the photosynthesis rate decreasing detectable green biomass by reducing the reflectance of Red-Edge/infrared region[14] [15]. Hyperspectral sensors usually are used when pigment degradation and structural changes happen, and we are interested in determining the most informative wavelengths to detect disease effects [16][17]. Additionally, hyperspectral imaging offers better opportunities for early detection and even discrimination of diseases than other methods. Changes in leaf structure and chemical composition of the tissues due to disease are highly pathogen-specific. Hence, the leaf spectral signature varies when infected by different pathogens. As a result, following the footprints can lead to the source pathogen. Several studies proved that hyperspectral scanning could distinguish different fungal infections[18]. Thermal cameras, on the other hand, can only discriminate plants that have a higher temperature for any reason including disease [19]. Other sensors, such as lidar, can be used to monitor diseases that affect plants' physical properties [20] and growth rate to indirectly correlate to long-term diseases, such as nematodes[21]. Sometimes, several data from different sensors are processed in unison to get as much data as possible. For instance, at [22] visible, infrared, and thermal camera used together to detect Huanglongbing in citrus. Table 4 shows two example of remote sensing studies for disease detection and control in nut orchards.
Table 5- Examples of remote sensing application for disease detection and control in walnut and almond.
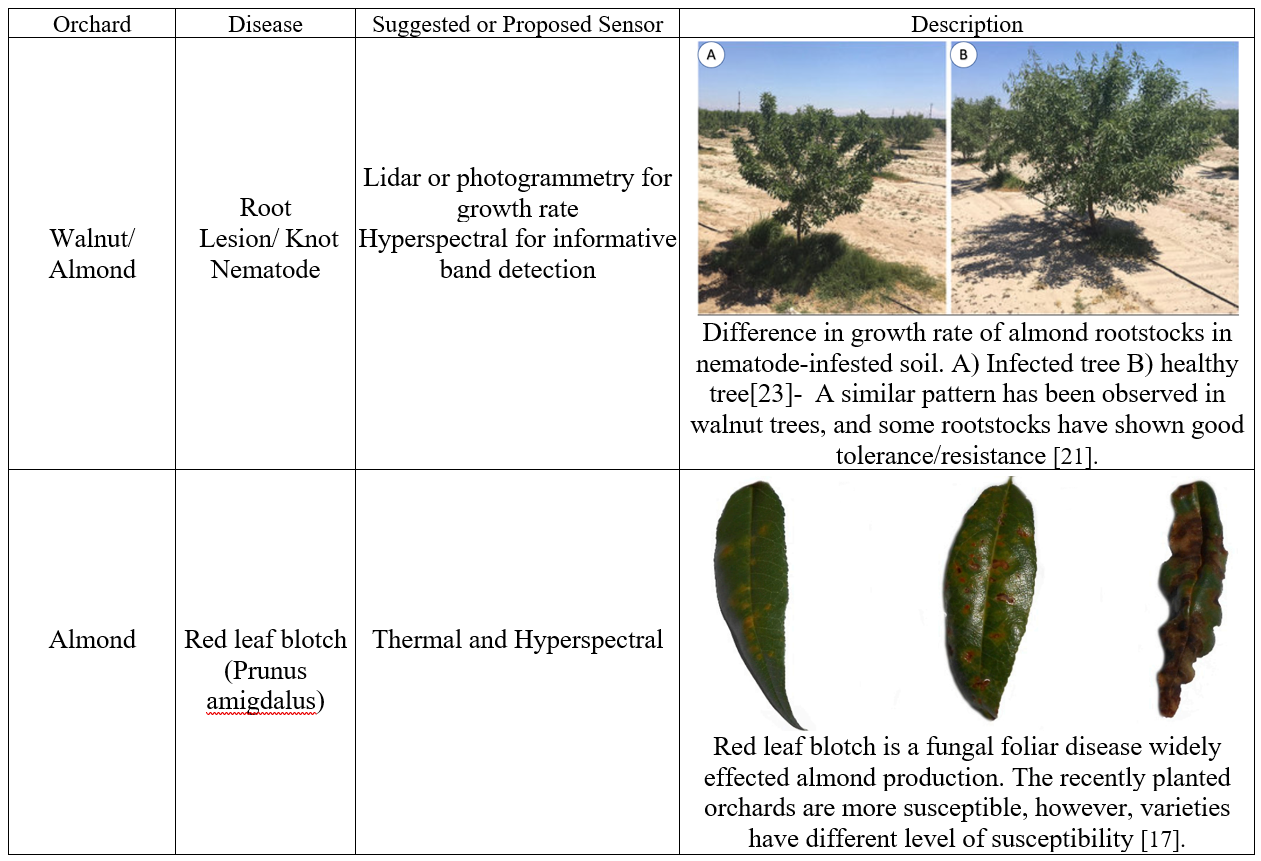
References
[1] A. D. Timmerman and K. Korus, “Introduction to Plant diseases,” the board of Regents of the University of Nebraska, 2014, [Online]. Available: https://extensionpublications.unl.edu/assets/pdf/ec1273.pdf
[2] G. B. Lucas and L. Campbell, Introduction to plant diseases: identification and management. Springer Science & Business Media, 2012.
[3] J. K. Brown, “Durable resistance of crops to disease: a Darwinian perspective,” Annual review of phytopathology, vol. 53, pp. 513–539, 2015.
[4] I. U. Haq, M. K. Sarwar, A. Faraz, and M. Z. Latif, “Synthetic Chemicals: Major Component of Plant Disease Management,” in Plant Disease Management Strategies for Sustainable Agriculture through Traditional and Modern Approaches, Springer, 2020, pp. 53–81.
[5] M. Lechenet, F. Dessaint, G. Py, D. Makowski, and N. Munier-Jolain, “Reducing pesticide use while preserving crop productivity and profitability on arable farms,” Nature Plants, vol. 3, no. 3, pp. 1–6, 2017.
[6] J. A. Martinez, Natural fungicides obtained from plants. INTECH Open Access Publisher, 2012.
[7] A. Pourreza et al., “Spray Backstop: A Method to Reduce Orchard Spray Drift Potential without Limiting the Spray and Air Delivery,” Sustainability, vol. 12, no. 21, Art. no. 21, Jan. 2020, doi: 10.3390/su12218862.
[8] W. Aktar, D. Sengupta, and A. Chowdhury, “Impact of pesticides use in agriculture: their benefits and hazards,” Interdisciplinary toxicology, vol. 2, no. 1, pp. 1–12, 2009.
[9] The Code of Federal Regulations (CFR), “Electronic Code of Federal Regulations (eCFR)-Title 7: Agriculture -The National List of Allowed and Prohibited Substances,” Electronic Code of Federal Regulations (eCFR), Oct. 14, 2020. https://www.ecfr.gov/ (accessed Oct. 16, 2020).
[10] H. F. Avenot, D. P. Morgan, J. Quattrini, and T. J. Michailides, “Resistance to thiophanate-methyl in Botrytis cinerea isolates from Californian vineyards and pistachio and pomegranate orchards,” Plant Disease, vol. 104, no. 4, pp. 1069–1075, 2020.
[11] R. M. Strickland, D. R. Ess, and S. D. Parsons, “Precision farming and precision pest management: the power of new crop production technologies,” Journal of nematology, vol. 30, no. 4, p. 431, 1998.
[12] F. H. Iost Filho, W. B. Heldens, Z. Kong, and E. S. de Lange, “Drones: innovative technology for use in precision pest management,” Journal of Economic Entomology, vol. 113, no. 1, pp. 1–25, 2020.
[13] R. Sugiura et al., “Field phenotyping system for the assessment of potato late blight resistance using RGB imagery from an unmanned aerial vehicle,” Biosystems engineering, vol. 148, pp. 1–10, 2016.
[14] J. Albetis et al., “Detection of Flavescence dorée grapevine disease using Unmanned Aerial Vehicle (UAV) multispectral imagery,” Remote Sensing, vol. 9, no. 4, Apr. 2017, doi: 10.3390/rs9040308.
[15] C. H. Bock, G. H. Poole, P. E. Parker, and T. R. Gottwald, “Plant disease severity estimated visually, by digital photography and image analysis, and by hyperspectral imaging,” Critical Reviews in Plant Sciences, vol. 29, no. 2, pp. 59–107, Mar. 2010, doi: 10.1080/07352681003617285.
[16] R. Calderón, J. A. Navas-Cortés, C. Lucena, and P. J. Zarco-Tejada, “High-resolution airborne hyperspectral and thermal imagery for early detection of Verticillium wilt of olive using fluorescence, temperature and narrow-band spectral indices,” Remote Sensing of Environment, vol. 139, pp. 231–245, 2013.
[17] M. López-López, R. Calderón, V. González-Dugo, P. Zarco-Tejada, and E. Fereres, “Early Detection and Quantification of Almond Red Leaf Blotch Using High-Resolution Hyperspectral and Thermal Imagery,” Remote Sensing, vol. 8, no. 4, p. 276, Mar. 2016, doi: 10.3390/rs8040276.
[18] A.-K. Mahlein, “Plant disease detection by imaging sensors–parallels and specific demands for precision agriculture and plant phenotyping,” Plant disease, vol. 100, no. 2, pp. 241–251, 2016.
[19] R. Calderón, J. Navas-Cortés, and P. Zarco-Tejada, “Early detection and quantification of Verticillium wilt in olive using hyperspectral and thermal imagery over large areas,” Remote Sensing, vol. 7, no. 5, pp. 5584–5610, 2015.
[20] N. A. Husin, S. Khairunniza-Bejo, A. F. Abdullah, M. S. Kassim, D. Ahmad, and A. N. Azmi, “Application of Ground-Based LiDAR for Analysing oil palm canopy properties on the occurrence of Basal Stem Rot (BSR) Disease,” Scientific reports, vol. 10, no. 1, pp. 1–16, 2020.
[21] A. Westphal, “New Ways of Managing Plant-Parasitic Nematodes Under Study,” 2019. https://californiaagnet.com/2019/05/24/new-ways-of-managing-plant-parasitic-nematodes-under-study/ (accessed Dec. 12, 2019).
[22] S. Sankaran, J. Maja, S. Buchanon, and R. Ehsani, “Huanglongbing (citrus greening) detection using visible, near infrared and thermal imaging techniques,” Sensors, vol. 13, no. 2, pp. 2117–2130, 2013.
[23] A. Westphal, Z. T. Z. Maung, D. A. Doll, M. A. Yaghmour, J. J. Chitambar, and S. A. Subbotin, “First Report of the Peach Root-Knot Nematode, Meloidogyne floridensis Infecting Almond on Root-Knot Nematode Resistant ‘Hansen 536’ and ‘Bright’s Hybrid 5’ Rootstocks in California, USA,” J Nematol, vol. 51, pp. 1–3, 2019, doi: 10.21307/jofnem-2019-002.