Nutrient management
Nutrient management is the practice of balancing the nutrient demands of a crop with the available sources during the growing season, considering all the variations in the field, including soil, weather, climate, and crop varieties, to maximize nutrient efficiency while reducing the off-site transfer of nutrients[1]. The primary objective of nutrition management is to accommodate conditions that favor the growth of healthy trees capable of producing top-quality fruit in high quantities[2]. Even though it seems a simple task, nutrient management becomes challenging in practice due to the myriad of factors involved, sometimes factors with long-term effects or even contradicting factors. For example, controlling the runoff prevents the topsoil nutrients from being washed away; however, preventing excess water from exiting a field might increase the nutrient leaching problem, leading to even serious consequences[3]. As another example of nutrient management complexity, managerial measures , such as fertilizer applications or pruning, each year can affect the nutrient status in the following years, causing a cycling or residual nutrients state[4]. As a result, knowing the field's current condition with as many details as possible helps prepare a suitable comprehensive plan for managerial practices.
Conventionally, two data collection methods were used to obtain information from the orchards' nutrient status: soil sampling and leaf tissue sampling. Soil sampling is conducted either before planting the new orchard to determine the type and amount of lime required and the relative levels of minerals or in existing orchards to provide additional insight for interpreting leaf samplings[2]. However, studies found weak correlations between the results of soil and leaf samples in orchards. Low correlations might be due to shallow sampling depth, mis-selection sampling zones, and mobile nutrients that are stored in various parts of the trees. Additionally, soil sampling shows the "plant available" nutrients that are not necessarily uptaken by plants. As a result, the focus on leaf-based nutrient analysis and management increased, and some standards were developed in response to noticeable variations in the results of samples collected at different growth stages, with different methods, and with different sample preparation and analysis procedures[5] [2]. These efforts established the ‘Critical Value’ concept in which fertilizers are applied to ensure that leaf nutrient concentrations never fall below the previously established critical concentration associated with optimal yield levels for each plant[6].
Based on the critical value concept, at least 14 mineral elements are identified as required for adequate nutrition of plants; six macronutrients that plants need in larger quantities including, nitrogen (N), phosphorus (P), potassium (K), calcium (Ca), magnesium (Mg), and sulfur (S) and eight micronutrients that are consumed in smaller quantities including chlorine (Cl), boron (B), iron (Fe), manganese (Mn), copper (Cu), zinc (Zn), nickel (Ni) and molybdenum (Mo) [7]. Deficiency in any essential element will restrict the optimum tree function, and a severe shortage of an element presents some symptoms that will persist until the deficiency is compensated [8]. In addition to deficiencies, some other nutrient disorders and imbalances such as N/K imbalance are identified that can adversely affect plants' performance [9]. Even though the critical value concept has been used widely for diagnosing nutrient deficiency over the past decades, it is now recognized that this approach has serious shortcomings. Some examples of the reported shortcomings are as follows 1) yield-based critical values for most of the minerals/plants are not available, 2) The recommended values does not satisfy the current yield levels, 3) since the standards are based on the leaf samples in the beginning of summer, they are not useful early in the season[6]. Another fundamental inadequacy of the leaf sampling methodology is environmental concerns, especially regarding excess nitrogen application, leading to nitrate polluted underground waters. Subsequently, new legislations implemented requiring the growers to apply nitrogen to meet, but not exceed, the annual N demand for crop and tree growth and nut production[10].
To ameliorate the mentioned problems, recently, a nutrient budgeting based on I) the correct crop's demand (Right rate), II) accurate nutrient uptake time (Right time), III) proper place around the active root zone (Right placement), and IV) the correct form of the fertilizer i.e. fertilizer, humus, etc. ( Right source), has gained widespread acceptance that is also known as 4R practice[11], [12]. However, determining the correct Rs are challenging and needs constant monitoring of the plants and creating nutrient status map that would be very expensive with traditional methods[13].
Remote sensing has shown great potential in providing sought after data for nutrient management and addressing the barriers in site-specific management. For example, a survey in 2013 showed that satellite and aerial imagery for creating nutrient management zones and status maps increased from 31% in 2011 to 40% in 2013 [14]. Similarly, the Web of Science search results shows that nutrition-related papers in agricultural fields are more than doubled during the five years of 2016-2020 compared to the previous five years of 2011-2015.
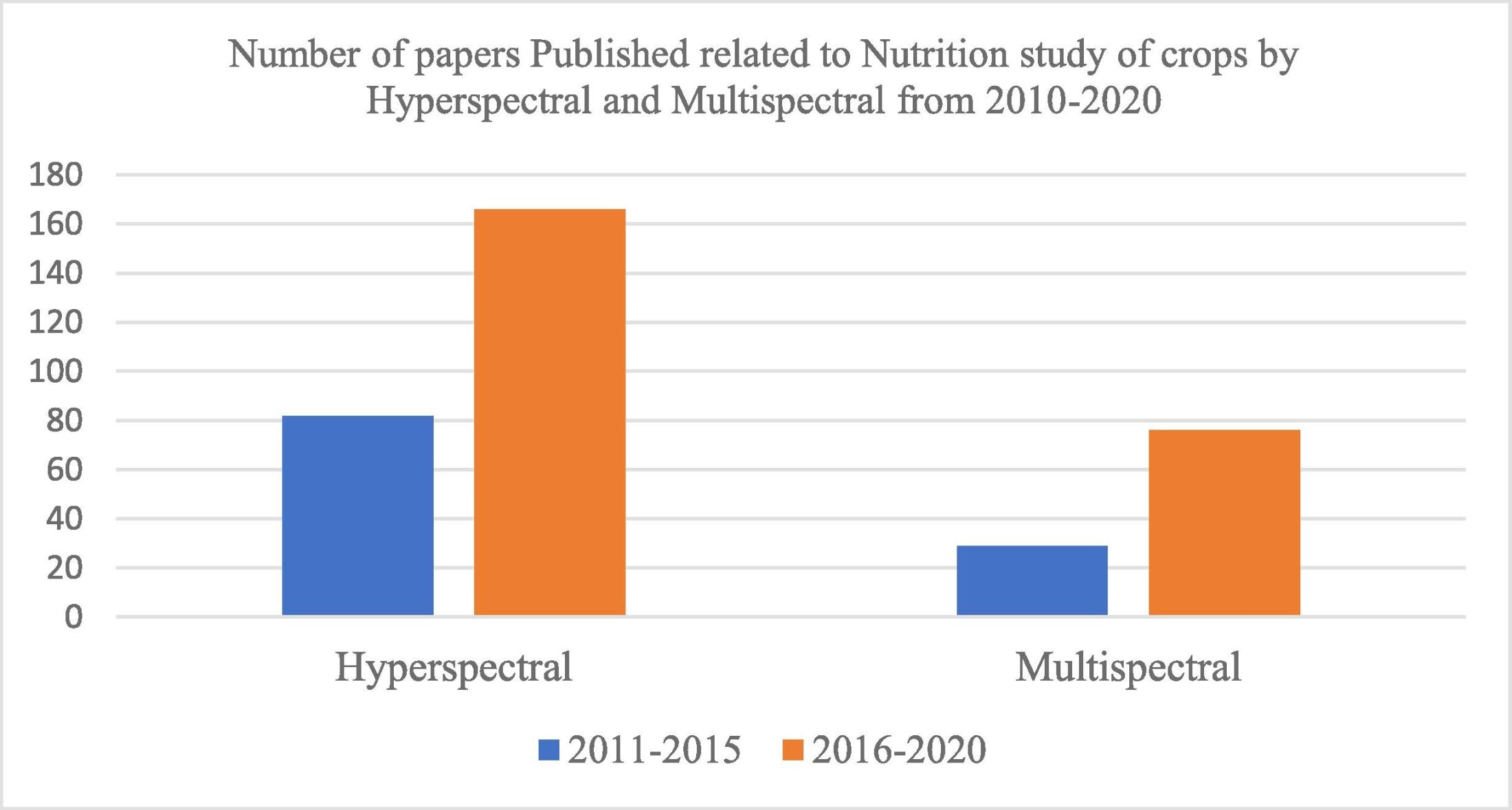
Figure 5 - The Web of Science search results from 2011 to 2020 for hyperspectral and multispectral aerial imaging. Results were retrieved by search keywords as following: 1) hyperspectral (nutrient OR nutrition) (plants OR crops) and 2) (multispectral OR multi-spectral) (nutrient OR nutrition) (plants OR crops)
Similar to other remote sensing techniques, the nutrient status estimation and management are mostly based on the plant's spectral reflectance. For a comprehensive comparison and explanation, refer to[15]. Most of the studies have tried to estimate the nitrogen content at a canopy-level using spectral vegetation indices[16] [17]. In contrast, as a new approach, some studies employed bands and wavelengths individually as a variable for predicting nutrient status, especially nitrogen[18] [19]. Studies showed that leaf nitrogen content can explain up to 76% of yield variation in almond orchards over two or three years[20]. However, we could not find any published research on nutrient status estimation by remote sensing techniques in nut trees, and this gap shows an opportunity for future research in this area.
References
[1] J. A. Delgado and J. Lemunyon, “Nutrient management,” in Encyclopedia of Soil Science, Marcel Decker New York, 2006, pp. 1157–1160.
[2] W. C. Stiles, “Orchard nutrition management,” 1991.
[3] N. Kiggundu, K. W. Migliaccio, B. Schaffer, Y. Li, and J. H. Crane, “Water savings, nutrient leaching, and fruit yield in a young avocado orchard as affected by irrigation and nutrient management,” Irrigation Science, vol. 30, no. 4, pp. 275–286, 2012.
[4] J. A. Delgado and J. Lemunyon, “Nutrient management,” in Encyclopedia of Soil Science, Marcel Decker New York, 2006, pp. 1157–1160.
[5] T. A. Obreza, M. Zekri, E. A. Hanlon, K. Morgan, A. Schumann, and R. Rouse, “Soil and leaf tissue testing for commercial citrus production,” University of Florida Extension Service. SL253, vol. 4, 2010.
[6] S. Saa, S. Muhammad, and P. H. Brown, “Development of leaf sampling and interpretation methods and nutrient budget approach to nutrient management in almond (Prunus dulcis (Mill.) DA Webb),” in VII International Symposium on Mineral Nutrition of Fruit Crops 984, 2012, pp. 291–296.
[7] P. J. White and P. H. Brown, “Plant nutrition for sustainable development and global health,” Annals of botany, vol. 105, no. 7, pp. 1073–1080, 2010.
[8] T. T. Obreza and K. T. Morgan, “Nutrition of Florida citrus trees,” EDIS, vol. 2008, no. 2, 2008.
[9] G. E. Shunfeng, Z. H. U. Zhanling, P. Ling, C. Qian, and Y. JIANG, “Soil nutrient status and leaf nutrient diagnosis in the main apple producing regions in China,” Horticultural Plant Journal, vol. 4, no. 3, pp. 89–93, 2018.
[10] Z. Zhang, Y. Jin, B. Chen, and P. Brown, “California almond yield prediction at the orchard level with a machine learning approach,” Frontiers in plant science, vol. 10, p. 809, 2019.
[11] S. Muhammad, S. D. S. Khalsa, and P. H. Brown, “Nitrogen Management in Citrus and Avocado,” The University of California, Division of Agriculture and Natural Resources (UC ANR), 2018.
[12] T. L. Roberts, “Right product, right rate, right time and right place… the foundation of best management practices for fertilizer,” Fertilizer best management practices, vol. 29, pp. 1–8, 2007.
[13] P. C. Robert, “Precision agriculture: a challenge for crop nutrition management,” in Progress in Plant Nutrition: Plenary Lectures of the XIV International Plant Nutrition Colloquium, 2002, pp. 143–149.
[14] B. Erickson, D. Widmar, and J. Holland, Survey: An inside look at precision agriculture in 2013. 2013.
[15] W. H. Maes and K. Steppe, “Perspectives for remote sensing with unmanned aerial vehicles in precision agriculture,” Trends in plant science, vol. 24, no. 2, pp. 152–164, 2019.
[16] L. Prado Osco et al., “Predicting canopy nitrogen content in citrus-trees using random forest algorithm associated to spectral vegetation indices from UAV-Imagery,” Remote Sensing, vol. 11, no. 24, p. 2925, 2019.
[17] L. P. Osco et al., “Improvement of leaf nitrogen content inference in Valencia-orange trees applying spectral analysis algorithms in UAV mounted-sensor images,” International Journal of Applied Earth Observation and Geoinformation, vol. 83, p. 101907, 2019.
[18] A. Moghimi, A. Pourreza, G. Zuniga-Ramirez, L. E. Williams, and M. W. Fidelibus, “A Novel Machine Learning Approach to Estimate Grapevine Leaf Nitrogen Concentration Using Aerial Multispectral Imagery,” Remote Sensing, vol. 12, no. 21, p. 3515, 2020.
[19] R. Omidi, A. Moghimi, A. Pourreza, M. El-Hadedy, and A. S. Eddin, “Ensemble Hyperspectral Band Selection for Detecting Nitrogen Status in Grape Leaves,” arXiv preprint arXiv:2010.04225, 2020.
[20] J. L. Zarate-Valdez, S. Muhammad, S. Saa, B. D. Lampinen, and P. H. Brown, “Light interception, leaf nitrogen and yield prediction in almonds: a case study,” European Journal of Agronomy, vol. 66, pp. 1–7, 2015.