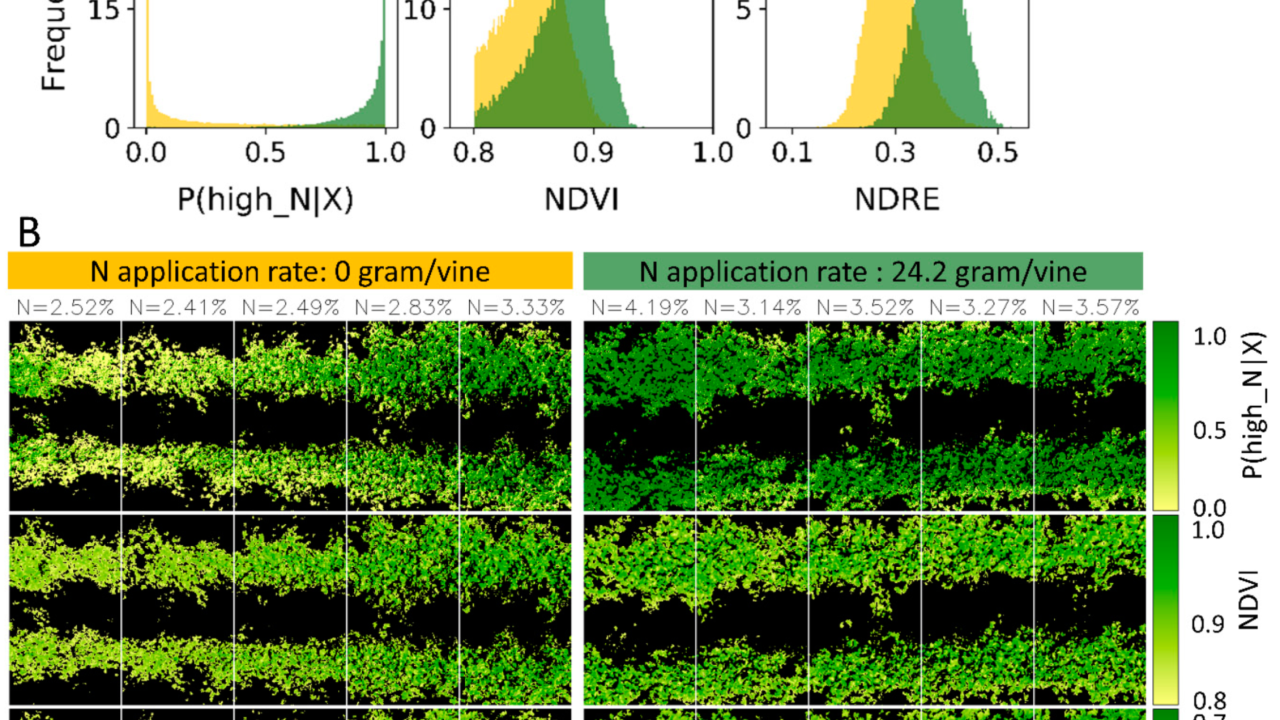
2019 HiRes Vineyard Nutrition Research Update
Estimating Grapevine Leaf Nitrogen Concentration Using Aerial Multispectral Imagery
Results published in:
Moghimi, A., Pourreza, A., Zuniga-Ramirez, G., Williams, L. E., & Fidelibus, M. W. (2020). A Novel Machine Learning Approach to Estimate Grapevine Leaf Nitrogen Concentration Using Aerial Multispectral Imagery. Remote Sensing, 12(21), 3515.
Our main objective in 2019 was to investigate nitrogen distribution within each vine further. We collected and assessed tissue samples from each vine (instead of combining 5 vines into one sample). Similar to 2018, we have 30 plots, each included 5 vines, and the lab analytics provided us with 150 nitrogen labels. In 2019 we had 5 times more labeled samples than in 2018, which would improve the N estimation model's robustness.
We collected very high-resolution multispectral imagery (altitude ~10 m) from each plot. The images were processed to segment each vine vegetation from the soil and adjacent vines. We used thresholds in NDVI and EGI indices for removing background pixels, then separated each vine using the markers we placed on the ground at the time of aerial imaging.
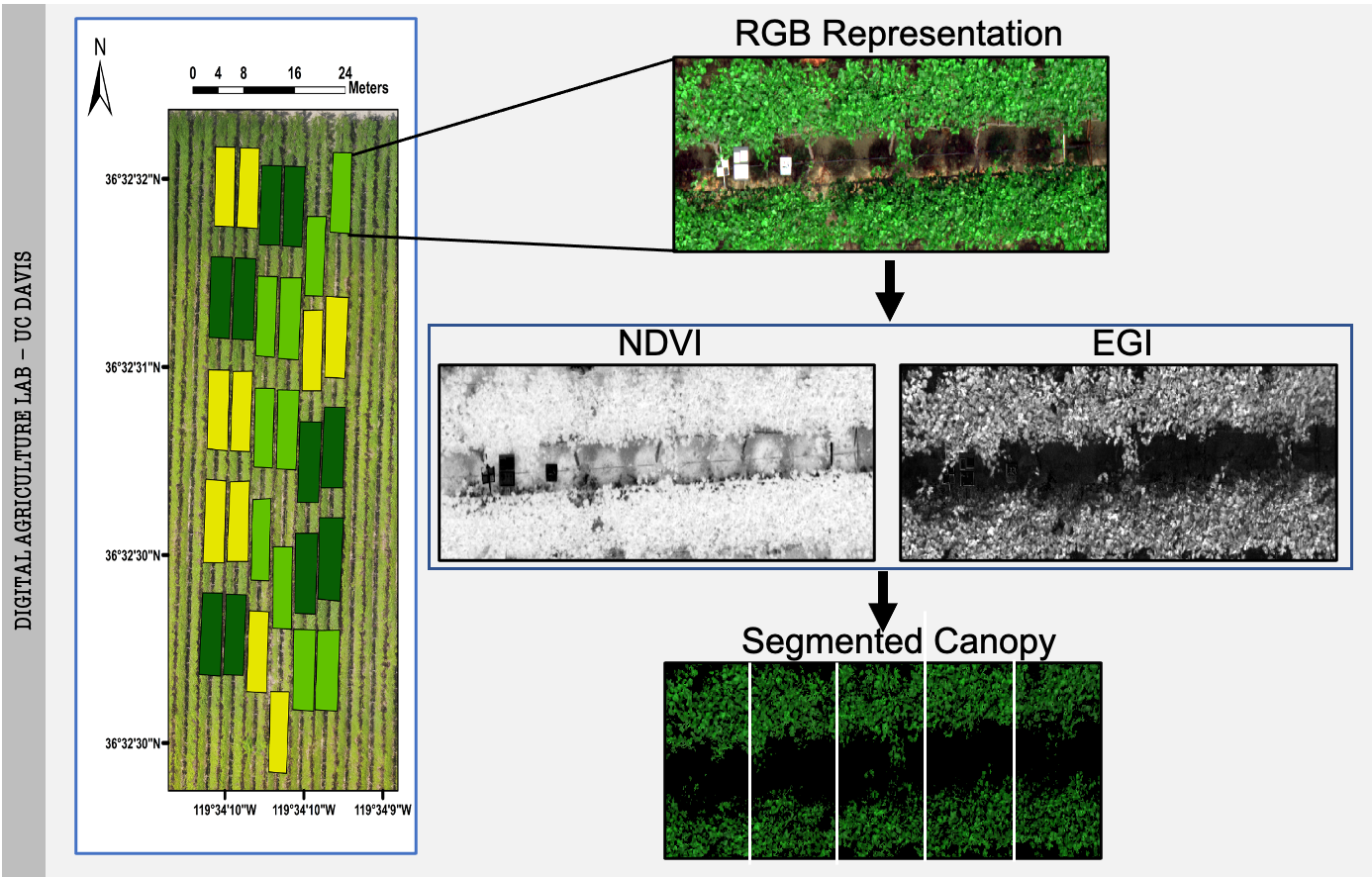
The following histogram shows the distribution of N content for the 150 vines in this experiment. This histogram suggests that N application rate is not necessarily correlated to nitrogen uptake in vine since we had N deficient vines that received maximum N application rate and vines with excess N that did not receive any N fertilizer.
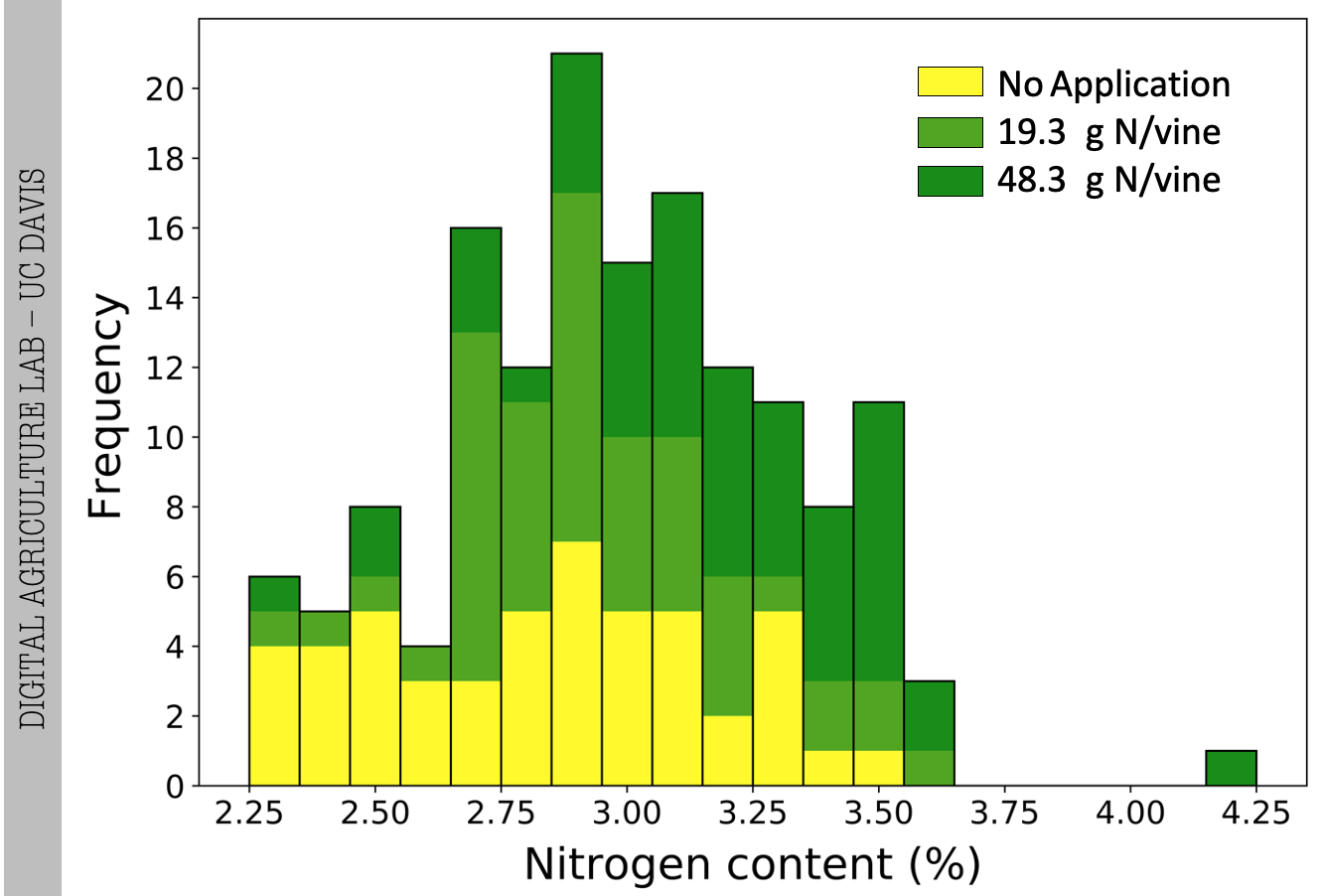
Using the N content labels, we trained several supervised learning methods with either excess N or extremely deficient samples. Then we tested these trained models with all other vines in the middle. The following figure shows the prediction results using the top two supervised learning methods: XGBoost, and Random Forest; both provided very similar R-squared and RMSE.
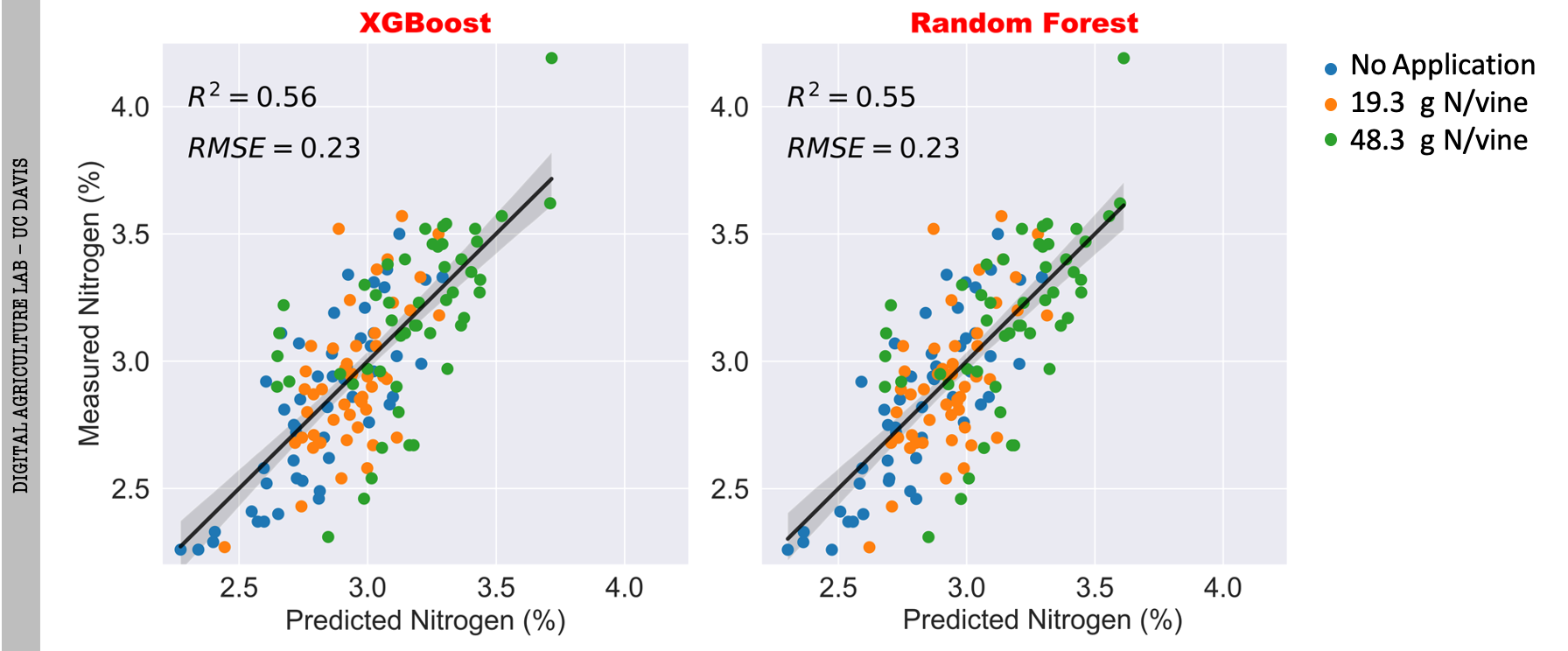
We compared the performance of a supervised-learning method and vegetation indices to identify N content and determine the spatial distribution of N in vines. The figure below shows the supervised-learning method's results [P(excess|X)] compared to NDVI and NDRE. In the top, the comparison of histograms of two vines (one deficient and one excess) shows a clear separation between the two vines, while in NDVI and NDRE, we see many overlaps of pixel values. Also, P(excess|X) clearly illustrates N's distribution at different parts of each vine, while these details are not visible in vegetation index representations.
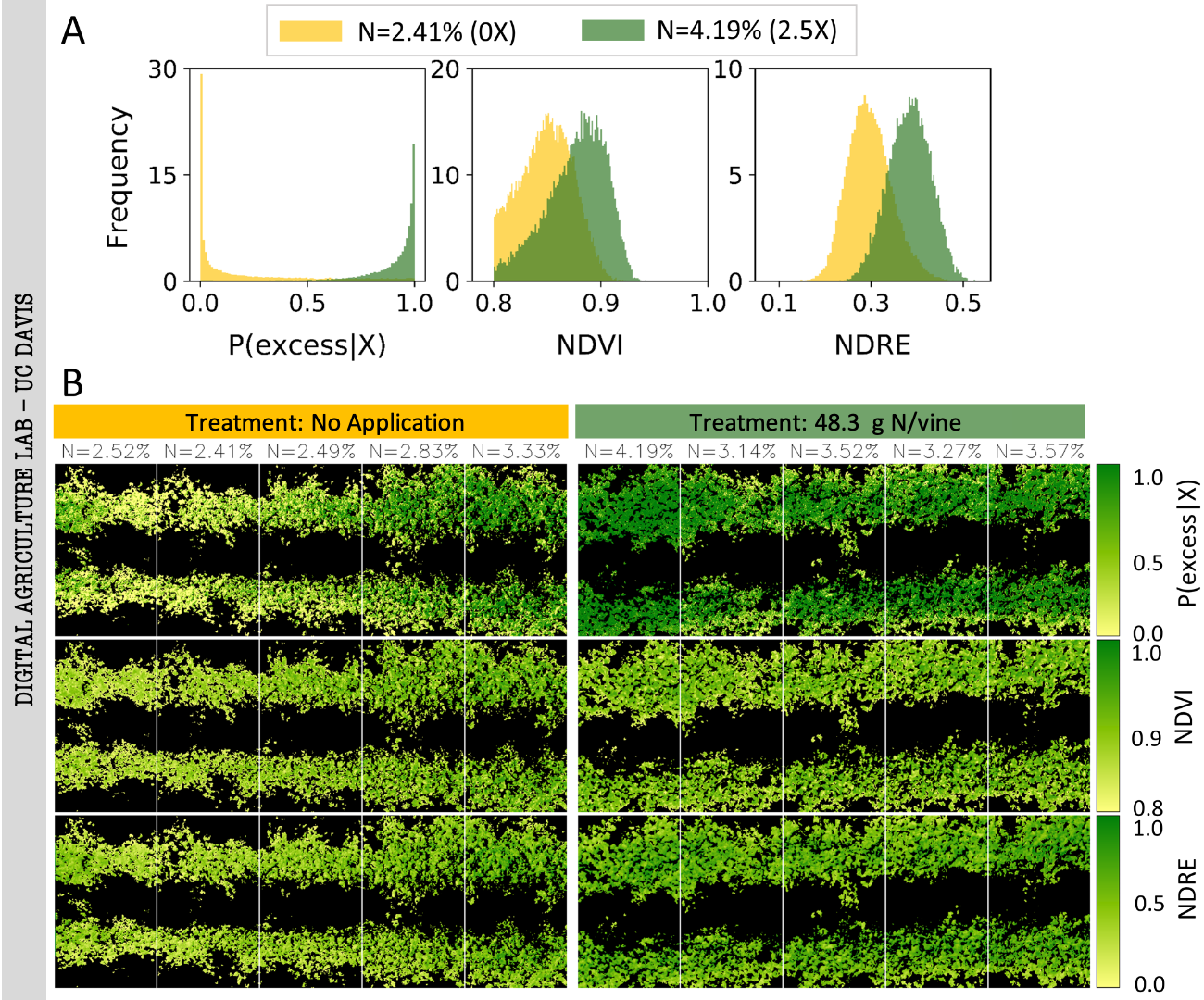