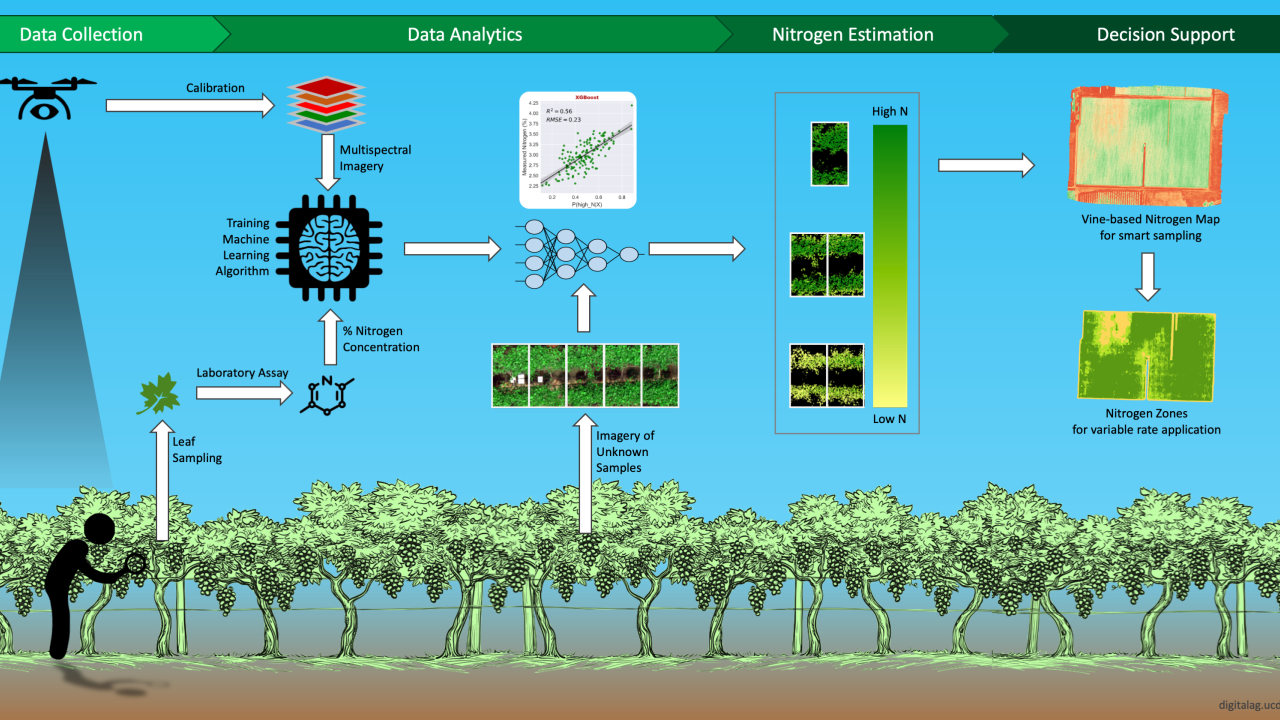
High-Resolution Vineyard Nutrient
Introduction
Future agricultural and food production systems must make better use of limited resources to ensure that farmers can economically produce more high-quality food for the growing world population while minimizing the environment's impact. Grapevines have lower mineral nutrient requirements than many other crops; still, vine nutrient status should be monitored to help prevent deficiencies that could reduce vine capacity, yield, and fruit quality. Monitoring nutrient status can also help growers prevent unnecessary fertilizer applications, which needlessly increase production costs and cause unintended nutrient imbalances or environmental contamination.
A nitrogen (N) management plan helps growers make the best use of N fertilizers, and an important part of such plans involves monitoring vine N status. Tissue sampling is one of the best ways to monitor nitrogen in grapevine. However, it is an imperfect monitoring method because it is based on a destructive, costly, and time-consuming laboratory assessment. This is a laborious practice that, and most of the time, provides an average value for the entire vineyard. In contrast, remote sensing can provide more detailed data about entire vineyards, potentially for every vine.
https://digitalag.ucdavis.edu/High-Resolution-Vineyard-Nutrient/2019
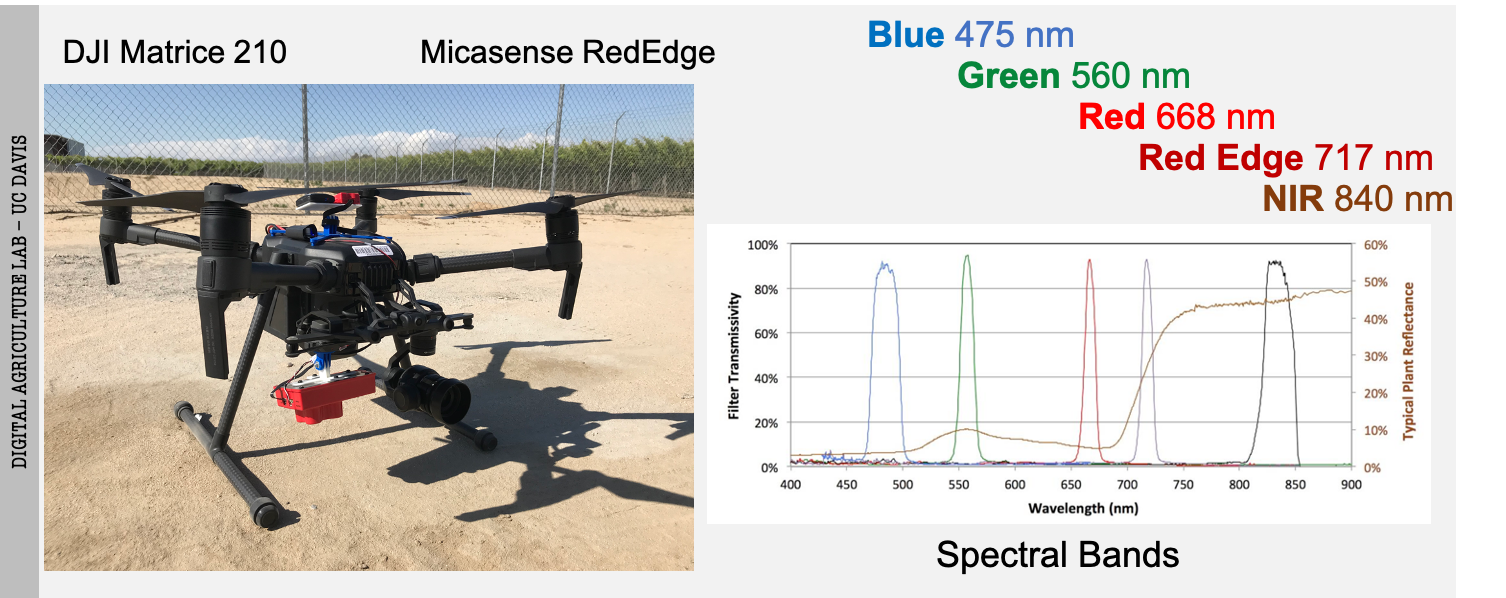
The recent introduction of sophisticated, more affordable, and user-friendly drone-based aerial imaging equipment has made it possible for growers to consider collecting frequent large-scale data for monitoring vineyards. However, a well-established aerial image processing pipeline, a comprehensive calibration procedure, and accurate data interpretation models are lacking. Until those exist, it will be difficult for growers to capitalize on aerial imaging applications fully. This project aims to develop a data-driven decision support tool that will enable growers to monitor vine nutrient status accurately. This tool will enable growers to use drone imagery to obtain site-specific insight about nutrient status throughout the entire vineyard. Over the last few seasons, we have been pairing aerial imaging and proximal sensing with laboratory analysis of tissue samples to develop calibrated models that should enable us to use remote sensing to estimate vine N status. The tools that will be developed in this study will be accessible via a web application that will automate most processing and data analytics steps and provide per-vine nutrient status at different stages of the growing season.
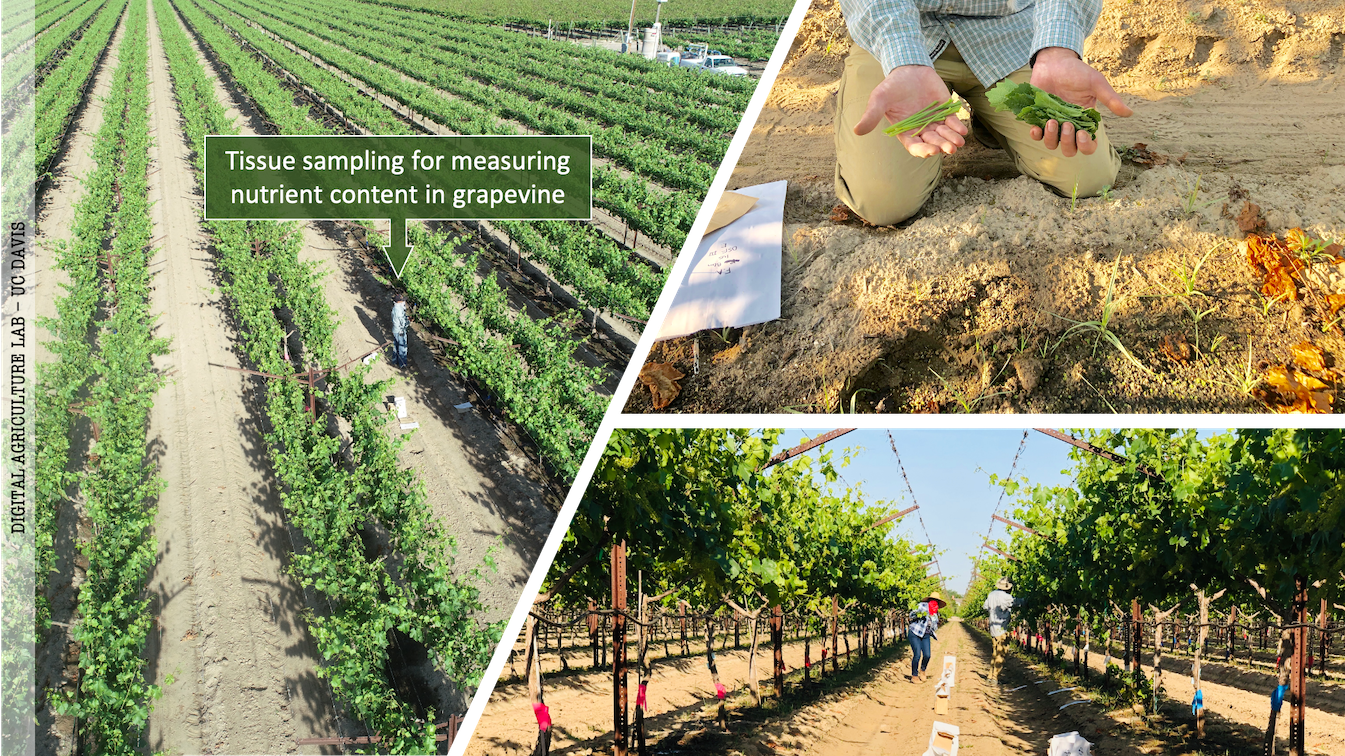
2021 Research Update
Our objective in 2020 is to understand temporal variabilities in aerial data and how they correlate to actual N content at three important stages of the growing season: bloom, version, and harvest. We are developing and calibrating a robust N content estimation model that can be practically used by vineyard managers.
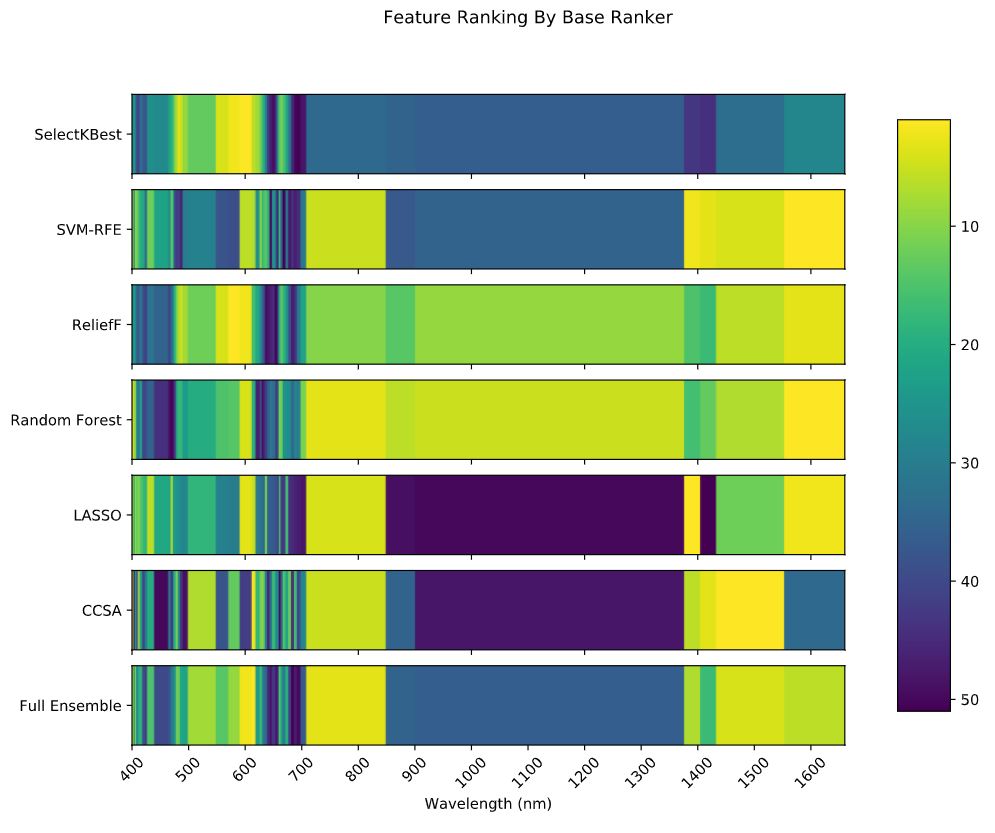
2020 HiRes Vineyard Nutrition Research Update
Selecting Optimal Multispectral Bands for Remote Sensing of Grapevine Nitrogen Using Hyperspectral Data
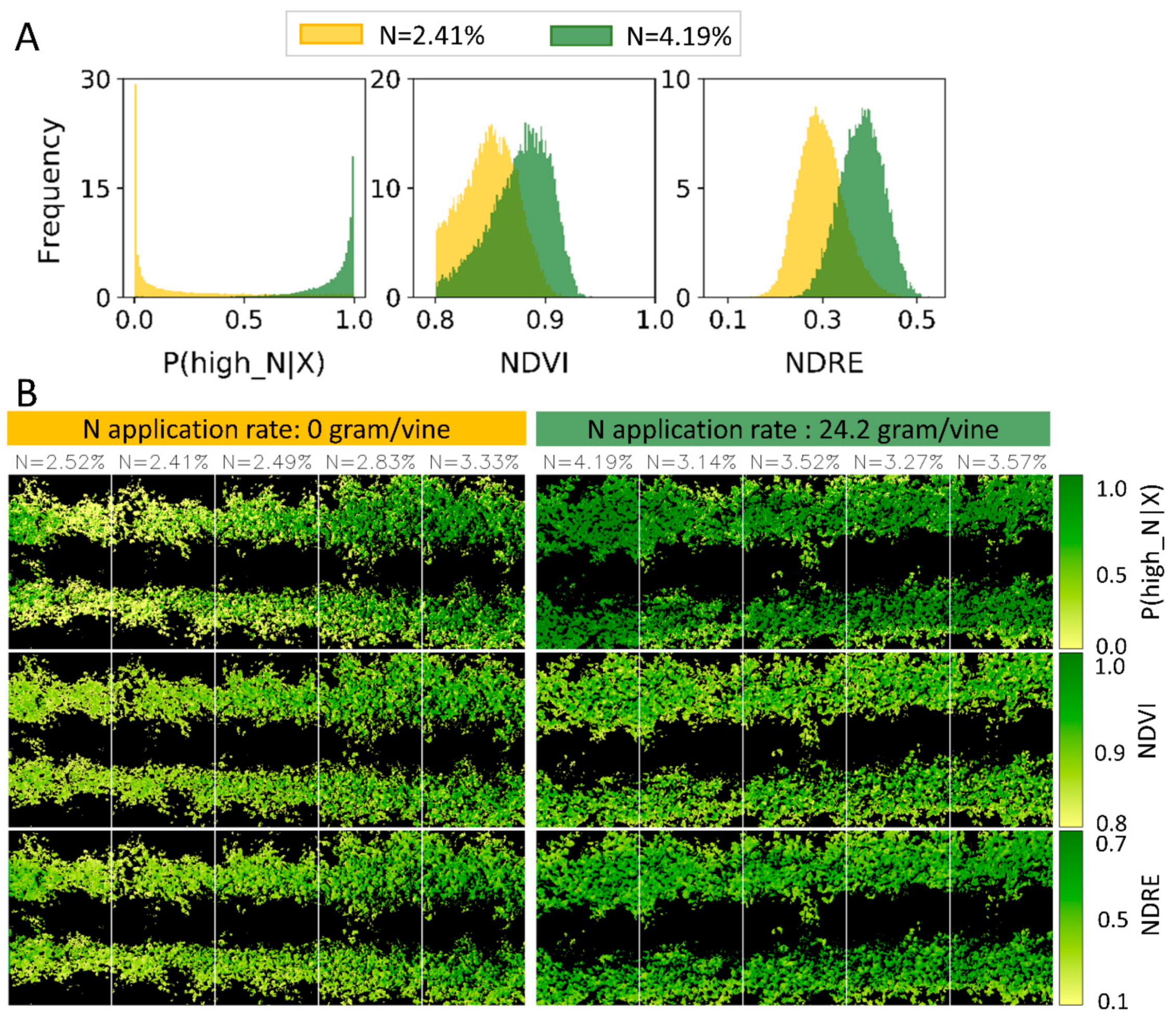
2019 HiRes Vineyard Nutrition Research Update
Our main objective in 2019 was to investigate nitrogen distribution within each vine further. We collected and assessed tissue samples from each vine. We collected very high-resolution multispectral imagery (altitude ~10 m) from each plot. The images were processed to segment each vine vegetation from the soil and adjacent vines.
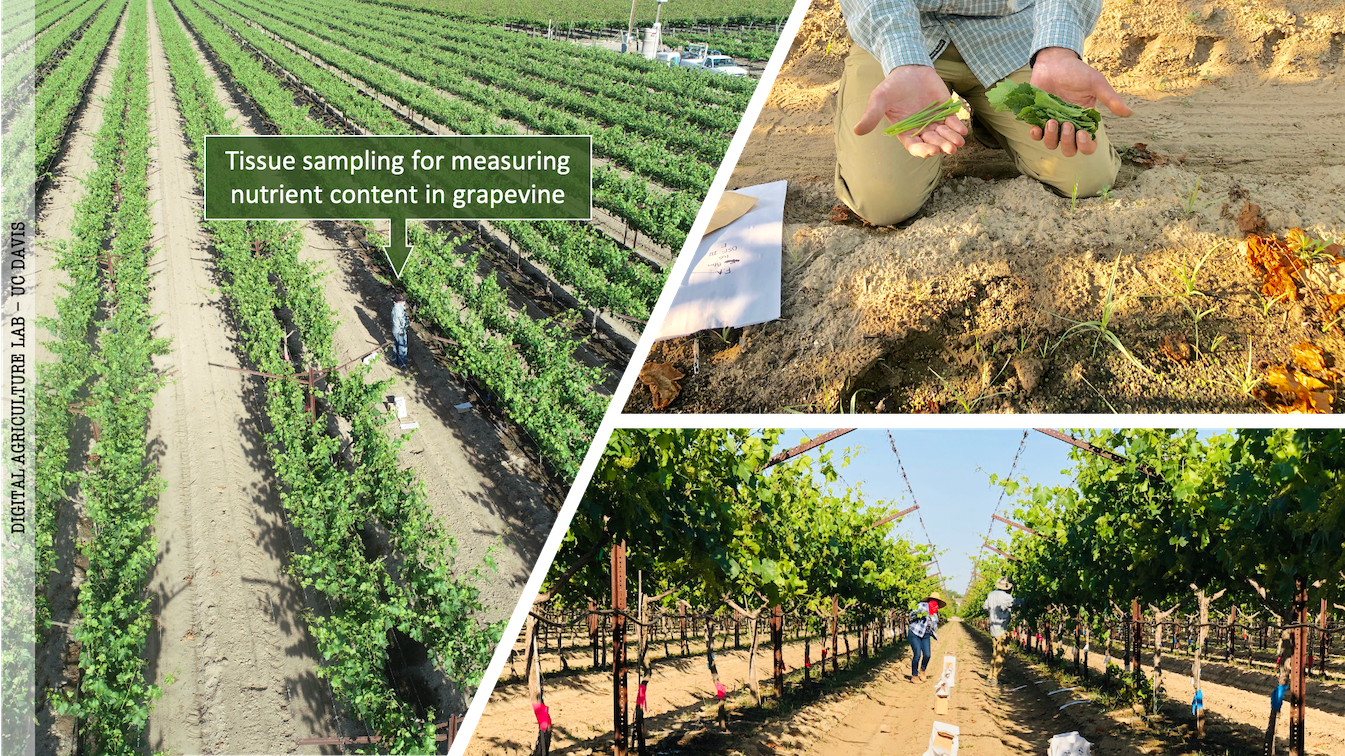
2018 HiRes Vineyard Nutrition Research Update
Our main objective in 2018 was to investigate aerial multispectral imaging's potential for detecting grapevine Nitrogen status. Out preliminary results confirmed that vineyard managers could obtain an insight into spatial variability of nitrogen throughout the entire vineyard using drone-based multispectral imagery.
Principal Investigator: Alireza Pourreza
Project Team: Ali Moghimi, German Zuniga-Ramirez, Ryan Omidi, Sirapoom Peanusaha, Yuto Kamiya
Collaborator: Matthew Fidelibus
Project Sponsor: California Table Grape Commission